Rough set-based classification systems [electronic resource] / by Robert K. Nowicki.
- 作者: Nowicki, Robert K.
- 其他作者:
- 其他題名:
- Studies in computational intelligence ;
- 出版: Cham : Springer International Publishing :Imprint: Springer 2019.
- 叢書名: Studies in computational intelligence,v.802
- 主題: Rough sets. , Computational Intelligence. , Artificial Intelligence.
- ISBN: 9783030038953 (electronic bk.) 、 9783030038946 (paper)
- URL:
點擊此處查看電子書
電子書(校內)
- 一般註:Introduction -- Rough Set Theory Fundamentals -- Rough Fuzzy Classification Systems -- Fuzzy Rough Classification Systems -- Rough Neural Network Classifier -- Rough Nearest Neighbour Classifier -- Ensembles of Rough Set-Based Classifiers -- Final Remarks. E1084學校採購電子書
-
讀者標籤:
- 系統號: 000274369 | 機讀編目格式
館藏資訊
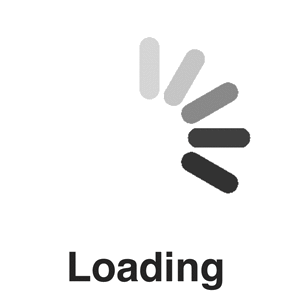
This book demonstrates an original concept for implementing the rough set theory in the construction of decision-making systems. It addresses three types of decisions, including those in which the information or input data is insufficient. Though decision-making and classification in cases with missing or inaccurate data is a common task, classical decision-making systems are not naturally adapted to it. One solution is to apply the rough set theory proposed by Prof. Pawlak. The proposed classifiers are applied and tested in two configurations: The first is an iterative mode in which a single classification system requests completion of the input data until an unequivocal decision (classification) is obtained. It allows us to start classification processes using very limited input data and supplementing it only as needed, which limits the cost of obtaining data. The second configuration is an ensemble mode in which several rough set-based classification systems achieve the unequivocal decision collectively, even though the systems cannot separately deliver such results.
摘要註
This book demonstrates an original concept for implementing the rough set theory in the construction of decision-making systems. It addresses three types of decisions, including those in which the information or input data is insufficient. Though decision-making and classification in cases with missing or inaccurate data is a common task, classical decision-making systems are not naturally adapted to it. One solution is to apply the rough set theory proposed by Prof. Pawlak. The proposed classifiers are applied and tested in two configurations: The first is an iterative mode in which a single classification system requests completion of the input data until an unequivocal decision (classification) is obtained. It allows us to start classification processes using very limited input data and supplementing it only as needed, which limits the cost of obtaining data. The second configuration is an ensemble mode in which several rough set-based classification systems achieve the unequivocal decision collectively, even though the systems cannot separately deliver such results.